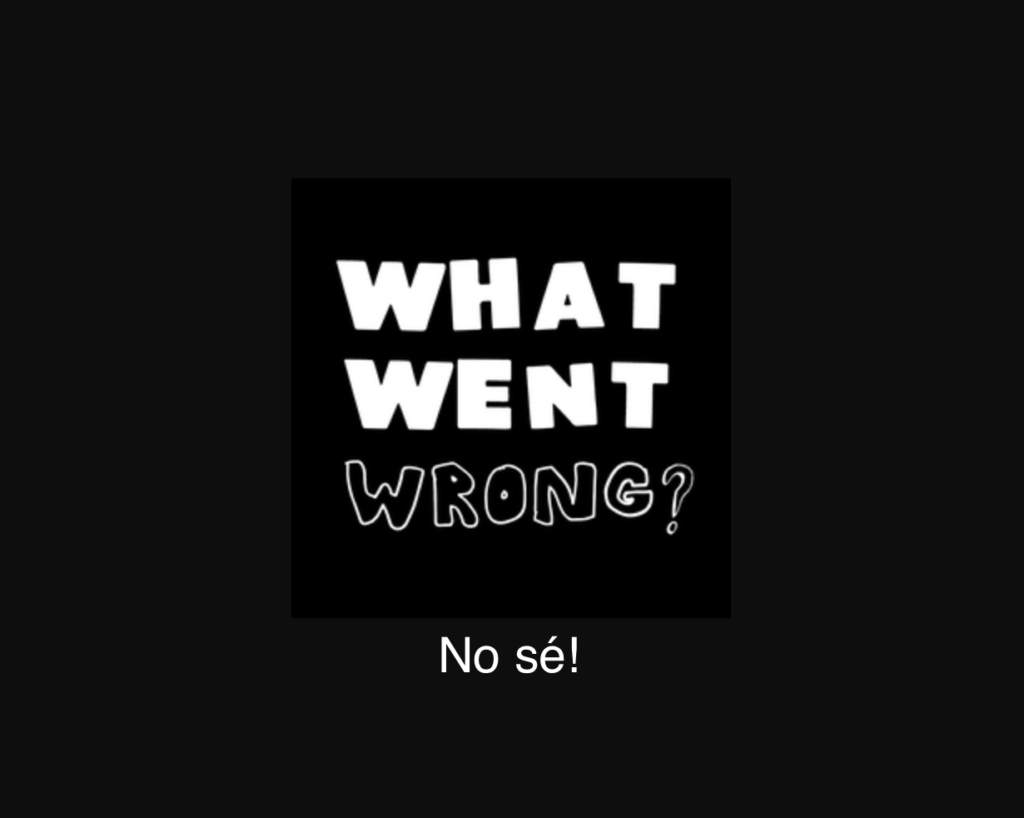
It is absurd when people use “data-driven” and it sounds like they meant “reliable” or “this is it, we’ve figured it all out”. In reality, we’ve been slowly waking up to the fact that machine learning and AI are actually far from being reliable, objective, or as good as it would be expected at this stage, especially when looking back over the last few decades and all the hype that’s gone into it. There are obviously pros and cons to data-driven technologies and approaches, like with everything else, but some of the issues (not only technical, but also ethical, social, and environmental issues) might just be too much of a hurdle to overcome. I personally love anything remotely related to playing with data, but like many others, I’m a bit disappointed with the state it is in at the moment, and how it’s been frequently going off the rails.
At the most basic level, the main task of data-driven approaches is to make predictions, based on some input data. As a consequence, some of these predictions will be necessarily wrong. How many? That depends on a very large number of factors, including in relation to the data (amount, quality, complexity, preparation, bias, concept drift, covariate shift, etc.) and the type of the selected algorithm and its parameters (whether in deep learning or machine learning). The fact of the matter is, nobody fully understands how all of these factors interact, and what’s more, nobody is reasonably able to fully test for it in the real world, as in how to come up with the perfect mix for a specific dataset or domain area (and how long that’ll last!). Now, it is rather accepted that these systems will always make inaccurate decisions, which, depending on the domain area it is operating in, might have serious consequences on the livelihood of people that depend on it. Consider AI-based diagnostic or recruitment systems for instance, and how inappropriate diagnosis or a biased hiring tool could affect people’s life.
Another issue that’s related to inaccurate predictions is that you cannot usually get clear evidence on what caused them. You can only give it a few guesses (hopefully as educated as possible), then go back and hope that’ll fix it. In terms of explainability or liability that’s obviously not ideal. From experience, it is effectively impossible to say for sure what’s driving a specific behavior, or in the case of errors, if it is a specific aspect that caused it (such as the abovementioned factors), or if it’s anyone’s negligence in particular (if we’re talking about liability). This has been exasperated over the years with deep learning, and although there are what’s called explainable or interpretable models, explainability is usually constrained by the size and complexity of the problem at hand. Maintaining a good level of explainability might not be always possible. Add to that the prospect of ‘adversarial attacks’ and it’ll take away any faith you had left. I’m not someone with trust issues, but I’m a sceptic and my scepticism about data-driven systems has grown over the years. I’m fine with Amazon recommending books but I would really have a hard time voluntarily trusting decisions from an AI-based system for anything that can remotely affect my life.
Another big issue with these systems is that they haven’t evolved to the point where they can understand and encode multiple (and possibly incompatible) ways to define what’s reasonable or fair, take into account the context of the data or the problem at hand, or more broadly local culture or even regulatory requirements. They might be good at some specific tasks, but reasoning, comprehension, or general intelligence, that’s tricky. This means we should avoid directly relying on these systems, especially for anything that affects human lives, and focus more on collaboration efforts; the human-in-the-loop thingy. Allowing feedback from humans, however, will only allow AI to adjust its view/ understanding of the problem at hand, but will not be able to fix the general issue of more realistic learning. The existing learning paradigms are simply not enough for that.
When ‘data-driven’ goes wrong…
Here are some examples of real-world data-driven systems going wrong. The tip of the iceberg!
- Amazon’s “holy grail” recruiting tool was actually just biased against women
- AI tools take ‘shortcuts’ that could lead to medical misdiagnosis
- Google ‘fixed’ its racist algorithm by removing gorillas from its image-labeling tech
- Facebook Apologizes For Embarrassing Mistake Caused By A.I.
- Why deep-learning AIs are so easy to fool
- IBM abandons ‘biased’ facial recognition tech
- Facial recognition fails on race, government study says
- Recruiting AI systems under fire for excluding workers
- Millions of black people affected by racial bias in health-care algorithms