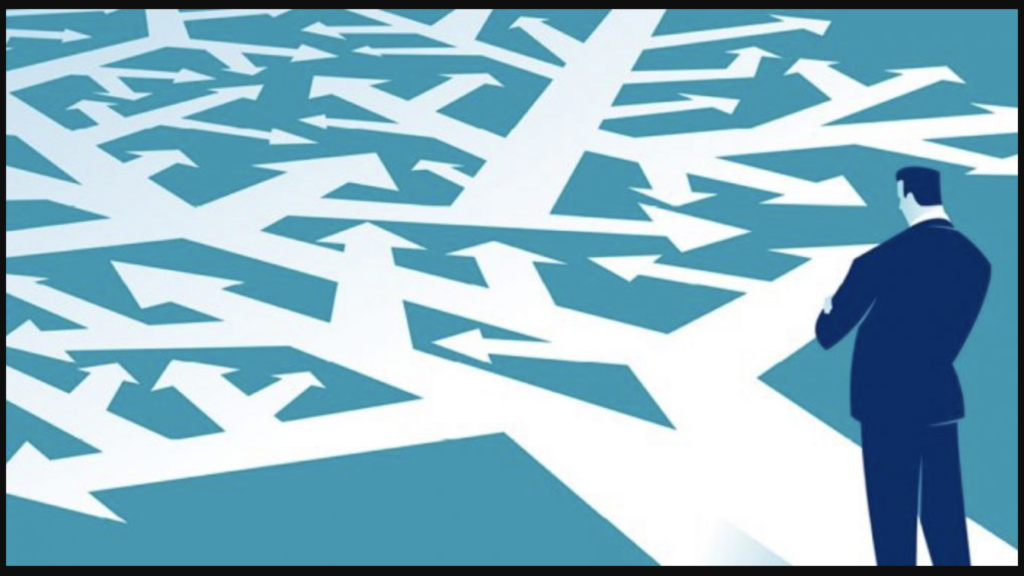
I’ve always been interested in arguments from the field of psychology that study how we make decisions, react, and are affected by a number of aspects, including biases (which I’ve talked about in a previous post). Choice overload is one of those cognitive aspects of decision-making that I was reminded of when I looked at the threat intelligence landscape a few months back, for the purpose of assessing threat intelligence sources out there. I realized then that a lot of people had jumped on that bandwagon. This isn’t unique in cybersecurity. Almost all areas of this field have a ridiculous amount of offerings and some sort of information overload. So what are the side effects of this overload?
The first one is ill-being! I haven’t read the book, but I have seen the TED talk of Barry Shwartz entitled ‘the paradox of choice’, where he described how we get overwhelmed by too many choices. Simply put, the more choices we have, the more miserable we are. Many others have also studied the effects of information overload and have even suggested that it might hurt our mental health and increase our vulnerability to stress and anxiety. However, since the level of happiness of cybersecurity analysts might not be the primary concern in our field, let’s talk about a couple of other aspects of overchoice and information overload, and why it is, for the most part, counterproductive.
No clear (or easily identifiable) differentiators
One might argue that “too many options” is a good problem to have, assuming you have the time and expertise to examine all potential technologies and technical solutions on the market. And even then, that would be the performance of a ‘default’ setup. Tuning a given technology to make it more effective, either individually or combined with other technologies, is another story altogether. Now, even under the assumption of efficient testing, to reduce the paradox of choice you still need clear differentiators, in the absolute, and some ‘guessing’ as well on how that could be helpful in your environment (starting off with a set of requirements and needs would then be necessary to have a clear understanding of a service unique value).
However, one of the issues in cybersecurity, including threat intelligence, is that the source of the data is often either vague or poorly described. Most vendors are understandably a bit secretive about certain aspects of their detection, generation, and collection processes (in order to protect their IP or access to third party data), but this makes things harder for the tester in mapping capabilities and requirements, and then, to identify a service differentiator and a potential unique value. An example of a source in threat intelligence that I found quite vague is the Dark Web. Also, details about processes (e.g. NLP) powering the collection and generation of data are rarely explained. These, and other sources and generation processes, should be described and categorized more granularly than just saying they exist.
The bottom line is, even with a clear security strategy, you can spend a huge amount of time assessing a significant list of vendors and end up with the wrong solution for you. A solution overview, or even a trial period, is not enough to allow you to fully analyze your options and distinguish between what is useful and what isn’t for your environment. Given the number of players and data involved, assessing the quality, coverage, and relevance of each vendor to establish clear differentiators will take months or years (and will likely change along the way!).
Cutting through the noise is far from trivial
There aren’t only too many vendors and solutions to choose from, there is also a market full of snake oil and a deluge of information that is increasingly difficult to filter out to a relevant, manageable, and digestible amount. What usually happens is that a lot of information is naturally going to be ignored, not necessarily because it’s irrelevant, but because decisions have to be made under time and resource constraints, and that’s how we can end up making truly bad decisions about important matters. Analysts use tools and/or custom automation as much as possible (using machine learning, regexes, whatever rules on things of interest), but it usually turns into a maintenance nightmare given how dynamic the landscape is. These get outdated quickly, and again, bad or uninformed decisions happen.
The noise is getting louder and louder by the day, generating a lot of distraction as well, and hence a lack of focus. The phenomenon known as ‘alarm fatigue’ or ‘alert fatigue’ is often brought up in cybersecurity. Analysts become desensitized to them and consequently miss important ones buried in a sea of data. Most organizations do not take the time to curate and analyze all detections and threat intelligence coming in for a reason – it will cost a lot (in terms of time and expertise) and you’re not even guaranteed a good outcome, so why bother. Our industry is contributing to its own problems by privileging quantity over quality, and taking the risk of perverse consequences of initially well-intentioned undertakings. If the industry doesn’t put in the effort to inverse this trend, including better ways to vet the data, I’m afraid it’ll eventually do more harm than good.